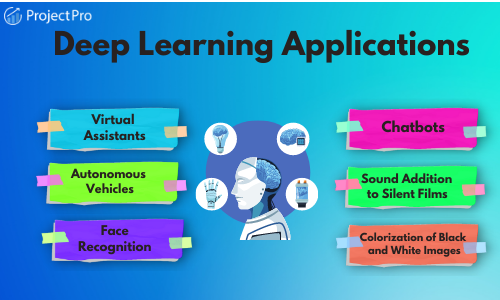
Computer Hardware for Deep Learning Applications
Introduction
Deep learning has become a prominent field in artificial intelligence, revolutionizing various industries. To effectively leverage this technology, it is essential to have computer hardware that can handle the intensive computational requirements of deep learning applications. In this article, we will explore the key components and considerations for selecting computer hardware for deep learning.
1. Graphics Processing Unit (GPU)
The GPU plays a crucial role in deep learning applications as it accelerates the training and inference processes. GPUs are designed to handle parallel computations efficiently and are well-suited for deep neural network operations.
When selecting a GPU, look for a model with a high number of CUDA cores and memory bandwidth. Additionally, consider the VRAM capacity as larger models or datasets may require more memory.
2. Central Processing Unit (CPU)
While GPUs handle the majority of deep learning computations, CPUs are still essential for overall system performance. CPUs are responsible for managing system processes, data preprocessing, and handling non-parallelizable tasks.
When choosing a CPU, prioritize higher core counts and clock speeds. Additionally, ensure compatibility with the GPU model to avoid potential bottlenecks.
3. Memory (RAM)
Memory plays a vital role in deep learning as it stores the models, data, and intermediate results during training and inference. Insufficient memory can lead to performance degradation or even failures.
For deep learning applications, opt for a higher memory capacity, ideally 16GB or more, to accommodate large datasets and models.
4. Storage
Storage affects data access and model loading times, impacting the overall training and inference performance. Choosing the right storage solution can significantly improve the efficiency of deep learning workflows.
Consider using Solid-State Drives (SSDs) as they offer faster read and write speeds compared to traditional hard disk drives (HDDs). SSDs are particularly beneficial in scenarios that involve large model sizes or frequent data transfers.
5. Cooling Solutions
Deep learning applications put immense strain on computer hardware, generating substantial heat during intensive computations. Adequate cooling solutions are essential to maintain hardware performance and prevent thermal throttling.
Investing in high-quality cooling solutions, such as liquid cooling or efficient air cooling systems, can help keep temperatures at optimal levels even during extended deep learning sessions.
6. Power Supply
Deep learning systems, especially those with high-performance GPUs, require a reliable and robust power supply unit (PSU). The PSU should have sufficient wattage to accommodate the power demands of the entire system, including the GPU and other components.
Ensure that the PSU is from a reputable brand and provides stable power delivery to avoid potential system instability or damage.
Conclusion
Choosing the right computer hardware is crucial for successful deep learning applications. GPUs, CPUs, memory, storage, cooling solutions, and power supply units all play significant roles in optimizing performance and achieving faster training and inference times.
Consider your specific requirements and budget when selecting computer hardware for deep learning, and ensure compatibility between the components to maximize efficiency and avoid potential bottlenecks.
Article written by [Your Name]